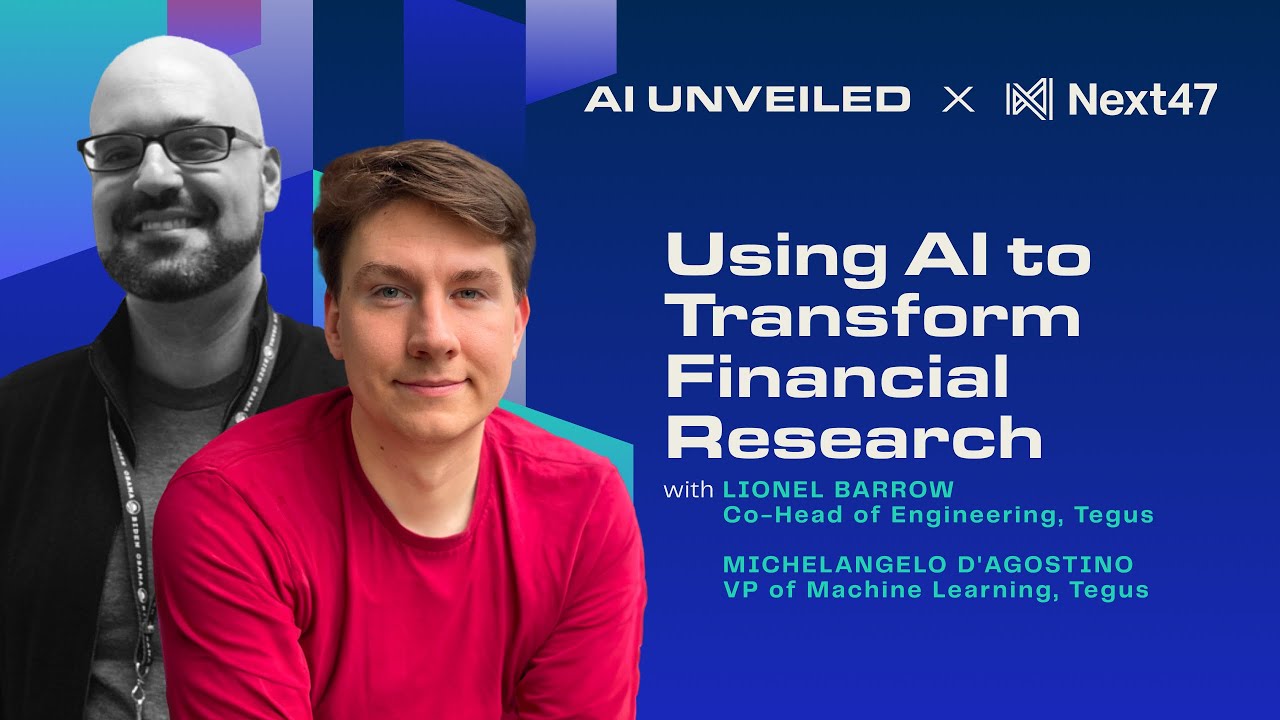
In this episode of AI Unveiled, Gaurav Kotak speaks with Lionel Barrow, Co-Head of Engineering, and Michelangelo D’Agostino, VP of Machine Learning at Tegus. Tegus is an investment research platform trusted by top hedge funds, PE, and VC firms. The platform includes over 60,000 expert transcripts, public company earnings calls, and presentations, as well as accurate financial models and KPIs.
They believe AI will bring a profound change in how financial research is done. From sifting through data to now surfacing insights based on past data, and where they are in the research funnel.
Both Lionel and Michelangelo are excited about the gold mine of data. The proprietary interview data on the Tegus platform is particularly interesting. In the interview, they note how important it is to balance fine-tuning or customizing the model with building an architecture that can easily plug in and avail of the latest foundational models.
They’ve started with a focus on applying AI to summarize and extract topics from specific calls, and are now adding AI-powered insights across an entire sector as well as automating part of their financial models.
We discuss how the company has different approaches for opinions (e.g. summary of a call) vs. facts (answering what revenue a company has)—whether it’s which models to use, how to assess quality, and when to rely on human-in-the-loop.
This episode provides a glimpse into the future of financial research. Enjoy!
Timestamps:
* 6:35 – How customer engagement evolves with AI
* 12:30 – Advice on tackling accuracy through product strategy and machine learning
* 25:21 – Incorporating human preferences into your AI
* 37:12 – Bringing AI into your org structure
Highlighted Excerpts
GAURAV: Can you elaborate a little bit more on how you manage accuracy? And how do you assess whether the accuracy is good enough for a specific use case?
MICHELANGELO: There is a new, interesting possibility that folks are using, which is using a language model to actually check the results of another language model. So can you use a powerful model like GPT-4 and run some documents through to generate a sort of golden set of labels that you then evaluate a second technique against? That’s sort of a new possibility.
But those sorts of models where it gets a little bit dicier are things like where you’re actually generating new pieces of text. So for example, if you’re generating a summary you’re answering a question or you’re paraphrasing something.
Those kinds of applications are a little bit harder to evaluate. And I think traditionally you might do something like have a human write a summary for something and then just count the overlap between what a human does and what the model does, but that’s really hard, and that doesn’t scale super well.
So the kinds of things that we’ve been doing internally are a mix of human evaluation like humans reading and rating a lot of these things internally. We’ve been trying to come up with clever sets of rules and checks that we can apply in code to various things. For example, if you think about summaries, you want to make sure that you’re not making up numbers—that any kind of number that it pulls out into a summary is a number that appeared in the transcript somewhere. And those are some kinds of things that you can code up checks against. So we have been applying techniques like that.
I think maybe the last thing I’ll say about the evaluation is you can evaluate as much as you want, but you need to give your customers the ability to follow up and evaluate as well.
LIONEL: I would say an interesting thing for us just from a product strategy perspective has been that it’s very nice to be able to be in a position where we kind of get a better mousetrap to play with like every month to a certain extent. I don’t know much, but I’m very confident that in a year from now, LLMs will be marginally more powerful or perhaps much more powerful than they are today.
And from our perspective, if we’re making products that are designed to give investors information or help them get information faster and faster—our position is quite nice because we are the originator of unique and proprietary and high-quality data. And so if we’re squeezing that data to get as much juice from it as possible—Sam Altman’s handing us a better juice squeezer every six months, and that’s very nice.
And ultimately we benefit and so do our customers. And so it’s good being in this position where you can focus on creating new experiences with new tools, but it’s backed up by the fact that you’re the originator of the data.
GAURAV: How do you measure the success of all the investments that you’re making in AI?
MICHELANGELO: The answer is a mix of both quantitative and qualitative user feedback. Our founders are very, very customer-focused. And every company says that they’re really customer-focused. But I haven’t worked anywhere where people are really as obsessed with talking to customers and spending time with customers as we are. A few weeks ago, I spent a week in Boston, just meeting with our customers, talking with them, and doing design exercises about potential ML features that we might build.
It’s our deeply ingrained company value to go out and talk to our customers. One good way we measure the success of these features that we’re building is to show them to our customers, get their qualitative feedback, introduce those quantitative feedback mechanisms, and just collect as many of those examples as possible.